- Admin
- January 24, 2025
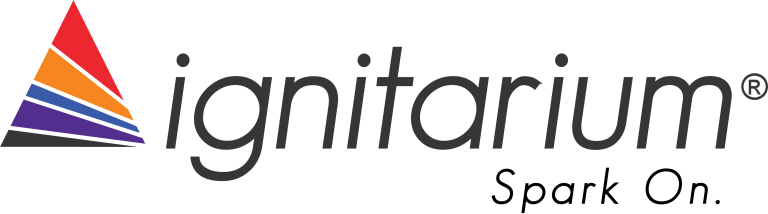
Case Study
Edge AI - GUI based No-Code AI Platform
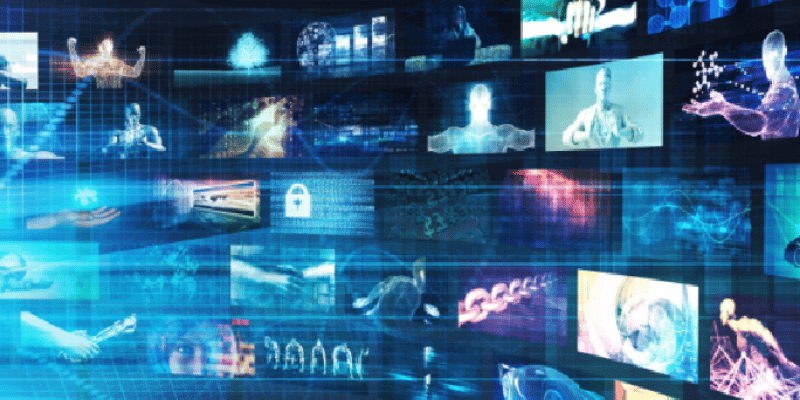
Client
A Tier-1 global semiconductor company that manufactures analog and embedded processing chips approached Ignitarium for an end-to-end AI / Deep Learning model development platform to help novice users train, compile and deploy such models on its embedded devices.
Scope
Define & develop an edge-to-cloud platform which enables user/s to capture, annotate, train, compile & deploy AI models to various edge devices
Challenges
While designing the solution, Ignitarium was required to address the following challenges:
• The platform was required to be deployed as a fully integrated no-code solution.
• In addition to deployment in the cloud, support for stand-alone deployment to customer labs.
• Simple interface and progress tracking to be provided for novice users to train and compile models for deployment into edge AI applications.
• Multi-tenant capability had to be built in for the platform with a constraint on resources availability for each user.
• Using the training and compilation software with pretrained models to train user’s own dataset by transfer learning and compile them for the customer’s various supported devices
• Providing accessibility to the edge device from a web browser with very low latency (2-3 seconds for live video streams).
• Integration and customization of the annotation tool to support the different types of labeling tasks.
Solution
• A fully integrated no-code multi-tenant platform for training & compiling models and deploying the same to customer-specific edge devices. It supports Bring-Your-OwnData (BYOD), enabling the re-training of models for the Image Classification & Object Detection tasks using customer proprietary models with custom data.
• Intuitive GUI to guide a novice user through the data capture, annotate, train, compile and model deployment steps on the supported embedded device connected via ethernet.
• Easy-to-read dashboard with the progress of the users' actions for training & compilation along with resource utilization.
• Support for accessibility to device cameras (through the web) for live data capture and inference.
• A unified development experience across customer’s multiple AI edge devices.
• Scalability achieved through Kubernetes deployment.
• Demonstration of the feasibility of the solution to the customer through rapid prototyping right at the project inception stage.
Business Impact
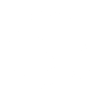
Reduced time to ‘Go live’ of the platform owing to collaborative delivery by our various teams across embedded, AI, UI / UX design and web development expertise
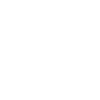
Enabled wider adoption of the customer’s edge devices in the market by addressing the needs of novice AI developers (users) through the platform