- Radhakrishnan V and Jeevanandham Duraisamy
- February 3, 2023
AI based Battery Management System (BMS) – Inference pipeline on Azure Cloud
Introduction
Batteries are at the heart of many modern electrical systems – Electric Vehicles (EV), Energy Harvesting Systems are some examples of such applications. In EVs, it is imperative that the driver is aware of the status of the battery powering his/her vehicle. For example, it may be important to know how long the vehicle can be driven before charging the battery. Likewise, knowing the remaining cycles (one cycle is typically a full charge and discharge) of the battery will assist the user in timely maintenance and to avoid downtime.
Battery Management Systems (BMS) are embedded in or alongside the battery packs driving these critical applications. BMS is a combination of electronics and software that monitors and manages electrical and thermal performance of rechargeable batteries, ensuring that they are within the safety margins specified by the manufacturers. It regulates the charging and discharging of batteries, thereby ensuring efficiency and longer life. BMS also provides vital statistics of the battery which can be used to calculate or predict the State of Charge (SOC), State of Health (SOH) and Remaining Useful Life (RUL).
An important area of research is in the use of Artificial Intelligence (AI) to make these predictions for the batteries used in automotive/energy harvesting systems. Ignitarium is developing AI models to predict SOC, SOH and RUL of batteries. These models must be plugged into a data pipeline capable of ingestion, processing and then storing the data for later use.
This blog outlines the steps to deploy a BMS inference pipeline on Microsoft Azure Cloud. Currently this article describes the inference pipeline using SOC prediction alone as the ML application; our SOH and RUL prediction AI models run on the same pipeline architecture. In the following sections, we will cover the architecture, the workflow involved, and the Azure services used in building and deployment of this solution.
Application
Deploying an AI based BMS solution needs careful consideration of several factors. Depending on the application, the solution should be capable of processing multiple input streams of battery parameters which could involve data processing, ETL (Extract, Transform & Load) functions, ML inference and visualization. Developing a custom solution for these functionalities would mean expending development resources on building and maintaining these components. We selected the Azure Platform to fast track the implementation of the inference pipeline.
Architecture and Workflow
Refer Architecture diagram in Figure 1 for workflow steps.
- Battery parameters from various BMS controllers are transmitted to Cloud Gateway devices over MQTT.
- Gateway devices send telemetry (raw data) to Azure IoT Hub.
- Azure IoT Hub feeds the data to Stream Analytics.
- Data Lake Storage receives data from Azure Stream Analytics job for long term storage and model training.
- Azure Stream Analytics job passes the message to a real-time prediction endpoint, which returns the prediction result.
- Azure Stream Analytics stores the prediction result in a database in Azure SQL Database for reporting.
- Power BI connects with SQL Database for reporting and visualization of historic predictions.
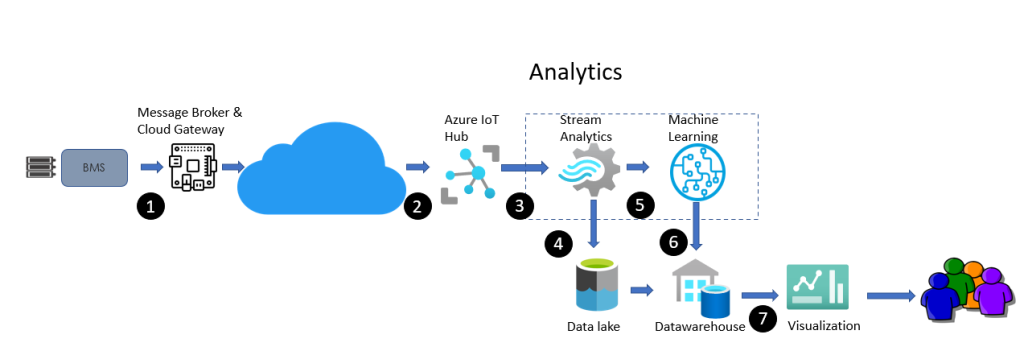
Components
- A Cloud Gateway controller act as the gatekeeper for multiple battery modules in the same site. Every 5 seconds, the modules push the monitored parameters which includes cell voltages, cell temperatures, charge and discharge values to the gateway controller using MQTT protocol. MQTT is a popular choice and widely supported in IoT communication. A connection string authorizes the gateway controller to push data to IoT Hub using HTTPS protocol
- Azure IoT Hub acts as a central hub facilitating communications between the devices and the hosted IoT application. IoT Hub can be integrated with other Azure services to build complete, end-to-end solutions.
- Azure Data Lake Storage is Azure’s implementation of Data lake. It stores the incoming data in its native format. Data lake stores are optimized for scaling to petabytes of data. It can store all types of data ( structured, unstructured or semi structured) from heterogenous sources.
- Azure Stream Analytics (ASA) is used to enable real-time and serverless stream processing. It connects to IoT Hub to source the input data and streams to the Machine Learning model for continuous inference. ASA can also embed some intelligence by filtering and/or transforming the input data it hands over to downstream components.
- Azure Machine Learning is a platform that facilitates rapid build and deployment of machine learning models. It provides users at all skill levels with a low-code designer, automated machine learning, and a hosted Jupyter notebook environment to support the preferred integrated development environment.
- A Data warehouse is implemented using Azure SQL Database, which is a fully managed relational database with built-in intelligence. For lighter workloads, this may be fine. If we have large number of devices connected, we must consider Azure Synapse Analytics (formerly Azure Data Warehouse).
- Microsoft Power BI Power BI is a unified, scalable platform for self-service and enterprise business intelligence (BI). Power BI can be connected to Azure SQL Server and leverage the real time data flow to visualize dashboards.
Challenges of on-premises data pipeline
On-prem implementation of this data pipeline using custom developed components would mean incurring technical debt. Our goal was to have a highly available and highly scalable solution, without the overheads of procuring, maintaining and monitoring the infrastructure.
Why Azure
Azure Cloud Services offered components that are not just easy to configure, but easy to scale the workloads. Azure’s platform provided a viable alternative and enabled rapid integration of pipeline components covering ingestion, storing data and serving real time predictions. There were challenges in exporting machine leaning model from the (on-prem) development environment to Azure Machine Learning Workspace. But this was quickly resolved, thanks to a plethora of support avenues.
Azure does provide multiple solutions at varying scales and cost. When the data velocity and volumes are low, the Data Lake component could be a simple Blob Storage. Similarly, we have implemented Datawarehouse using Azure SQL Database. But, if the number of connections and correspondingly, the data volume increase, Azure Synapse Analytics (Azure’s Data warehouse solution) should replace Azure SQL Database. This also enabled some cost saving without losing functionality.
Using all the components described, the ML-based battery-characteristics prediction pipeline was successfully implemented. Figure 2 is an excerpt from the dashboard which shows our ML-based model predicting the State of Charge of the battery module.
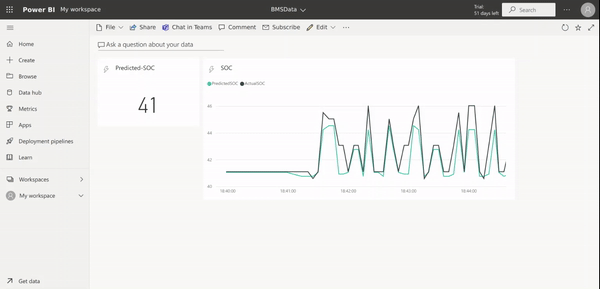
Infrastructure-as-Code
Foreseeing future deployment needs (deploying additional models, customer demonstrations, reliability and repeatability etc.), the deployment was converted to “Infrastructure as Code” using Terraform and Shell scripting. This enabled spinning up replicas of the existing pipeline in no time.
Conclusion
Azure IoT Hub is capable of handling multiple connections, hence connecting additional gateways was straight forward. No change was needed to the Azure components when we added a new gateway controller to the setup. Further, the architecture leaves scope to expand into newer services (for example, we can easily extend to battery parameter monitoring and setup threshold alerting just by plugging in Azure Notification Hub and setup triggers), thereby unlocking more value from the data.