- Abel David
- March 30, 2022
AI transforms driver safety and comfort: 4 things that will never be the same
Introduction
AI is increasingly becoming a part of our everyday lives, across different domains. Have you ever thought about the possibility of a custom driver and passenger experience based on your unique and personal needs? Will AI eliminate the need for us to drive altogether? Would it provide a convenient alternative to all our ‘on road woes’?
Right from prompts in the form of driver alerts on rash / distracted driving and driver drowsiness, to ensuring dynamic personalization of in-cabin preferences during the drive, to foreseeing system inconsistencies, AI is taking over multiple aspects of the experience of owning a car, from the basics of driving to the comfort of passengers in the vehicle. It all started with providing driver assistance for passenger and commercial vehicle segments, and then progressed into other areas, as we will see in this blog.
Let us close our eyes and imagine ourselves to be in a common driving scenario. You are changing lanes on the freeway, and suddenly, a blinking orange light on your side-view mirror and a loud beep alert you to a car coming alongside. This is an example of a blind spot detection feature, which is standard in vehicles with higher trim levels.
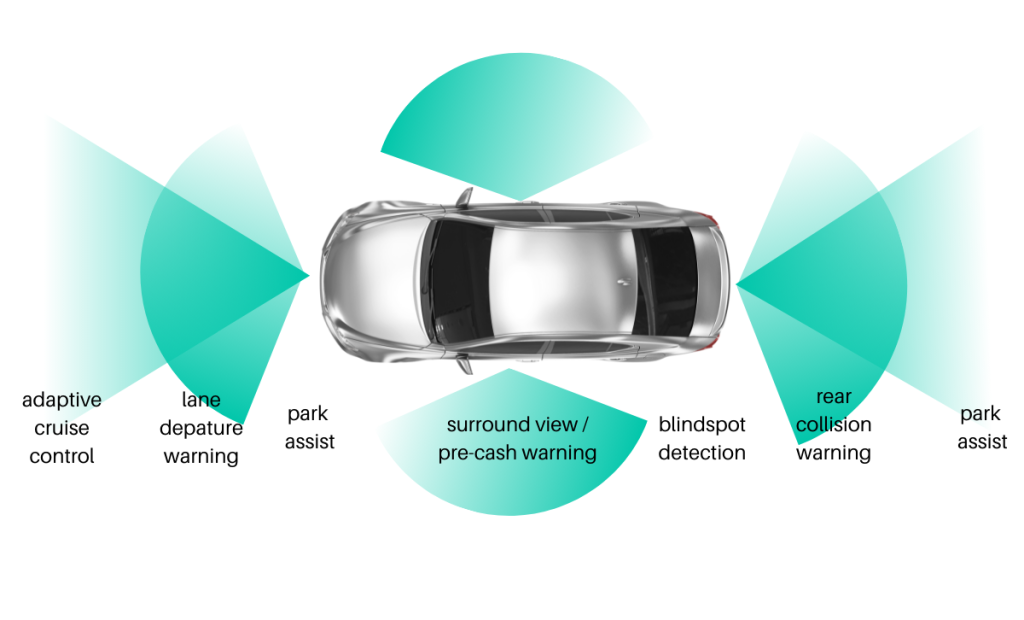
The automotive industry has started to incorporate more of such technology to keep drivers alert and accident-free. Multiple sensors and alert systems are used to help drivers prevent car accidents by alerting them about possible dangers, since most accidents result from human error. SAE’s Levels of Driving Automation, Level 01 & Level 02 – the global standard for driving automation lists out other features such as parking-assistance, lane-keep assistance, and Adaptive Cruise Control.
However, AI technology has not yet evolved to levels of full automation here. Instead, the driver is responsible for monitoring and reacting to their environment and therefore we require a system that checks the driver for high self-awareness levels. Let us take up a few AI and Deep Learning based automotive applications that enable these.
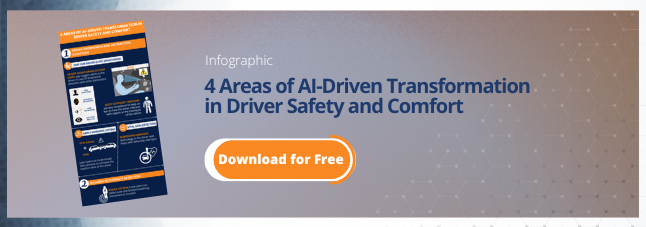
Driver drowsiness and distraction detection
What about all those driver distraction activities that are now becoming increasingly common, because of the devices in our hands and the added entertainment systems in the car? Often, we see drivers texting, speaking on calls, talking to passengers or busy shuffling music. While paying attention to these tasks, their attention tends to shift off the road. Here is where machine learning-based Driver Monitoring System (DMS) apps can be used to trigger alerts to the driver in case of drowsiness detection and other distractions as above.
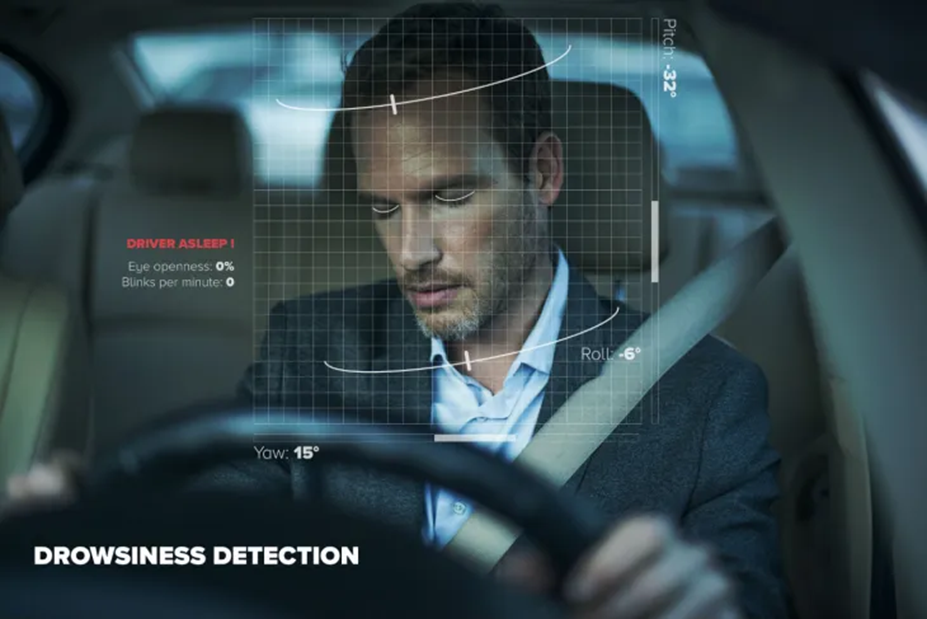
This is achieved with a mono camera facing the driver that streams video onto an AI-edge device that runs multiple convolutional neural networks (CNNs) for Face detection, Head pose estimation, Gaze estimation and Eye state analysis.
With the onset of Autonomous Driving (AD) features that are available in most of the higher-end trims, the car can cruise autonomously on the highway with a click of a button.
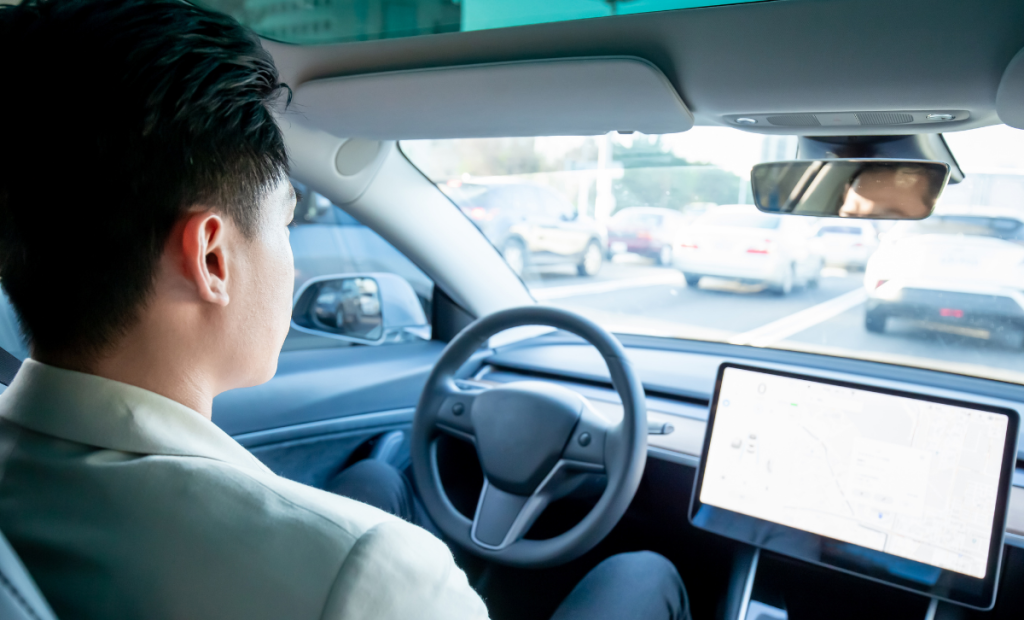
Once activated, the drivers’ hands are off the steering wheel. In this autonomous driving mode, Facial expression features alone cannot determine the inability of the driver to control the vehicle, when a sudden need arises. Moreover, the use of driver’s steering behavior over the course of long trips is also an indicator of the driver’s fatigue level and the onset of drowsiness.
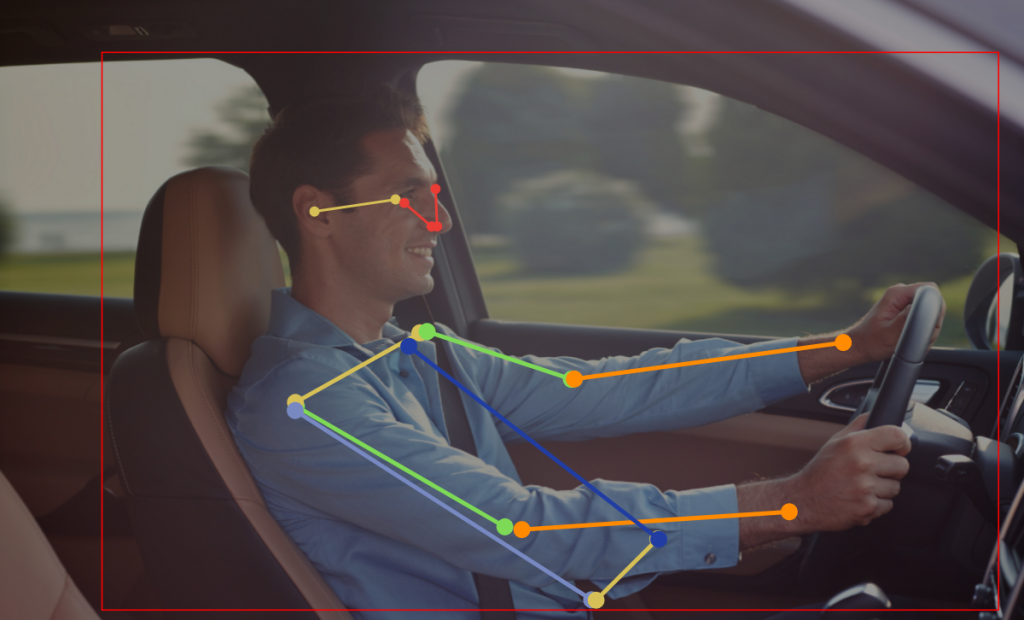
In the above case, CNN models for Body Keypoint tracking are also considered to keep an eye on how the driver interacts with objects or the interfaces of the vehicle.
Late fusion on multi-modal data streams from Forward Collision Warning (FCW) Radar with the in-cabin DMS camera is a more effective solution to increase the reaction time of the driver for different danger levels, compared to a scenario where there is only DMS detection in the absence of FCW.
Embedding mmwave radars in the driver seats also helps with detecting vital signs such as Respiration rate (RR), Heart rate (HR) and Heart rate variability (HRV). If the system identifies signs of unconsciousness from the heart rate variations of the driver, it alerts the vehicle occupants, and also the AD features kick in to bring the vehicle to safety.
In-cabin Occupancy Detection
With increasing automation and better connectivity, safety systems will continue to play a major role in the future. One way to improve safety in automobile and commercial vehicles is to install radar systems in the cabin that can detect even the faintest breathing movement of humans.
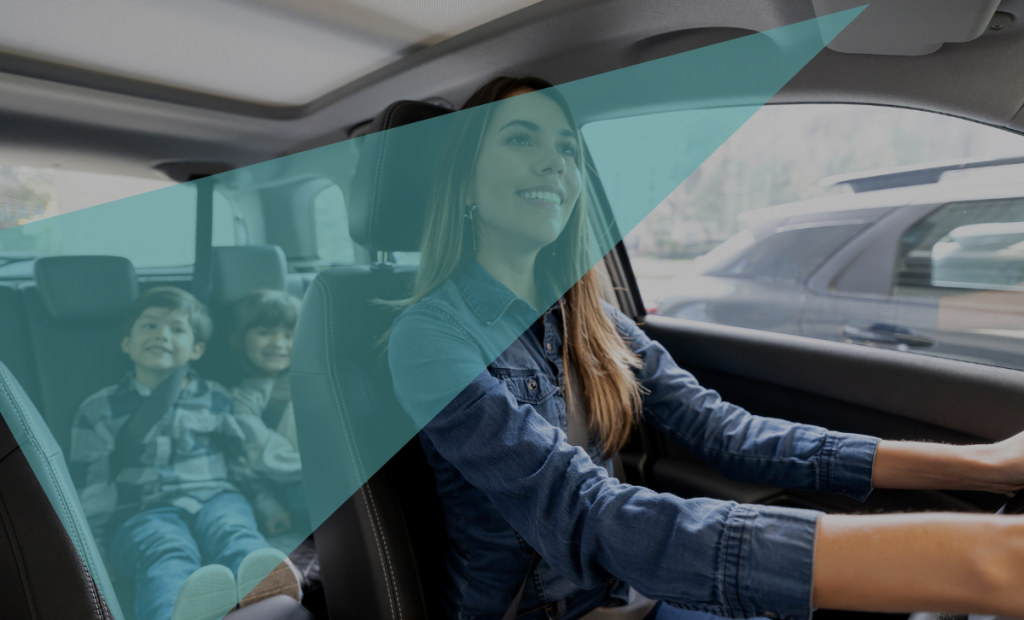
One critical use case is child occupancy detection, where children are inadvertently trapped or left unattended in vehicles, which can lead to heatstroke and other such casualties. With a proper safety system in place, if the child is left behind, the system notifies the vehicle, which then sends out warning signals, to get the attention of the car owner to act on time, and with urgency.
Another area of use is to extend occupancy detection to optimizing occupants against injuries in collisions including vehicle roll overs where, the signals from the sensors detect the strength and direction of a collision and can activate the restraint mechanisms in the vehicle, for example seatbelt pretensioners and airbags, as required for maximum occupant protection. These systems save lives and protect vehicle occupants from injury in case of a collision as it keeps the accelerations and forces in the event of an accident as low as possible.
In-cabin Noise Suppression
In fleet vehicles, where driver’s speech must be recorded or used to control devices, the ambient noise (from ICE engine or HVAC or wind) can result in degraded speech. For real-time noise suppression that counter stationary and non-stationary noises, traditional ANC methods which rely on generating anti-noise, do not fit the bill.
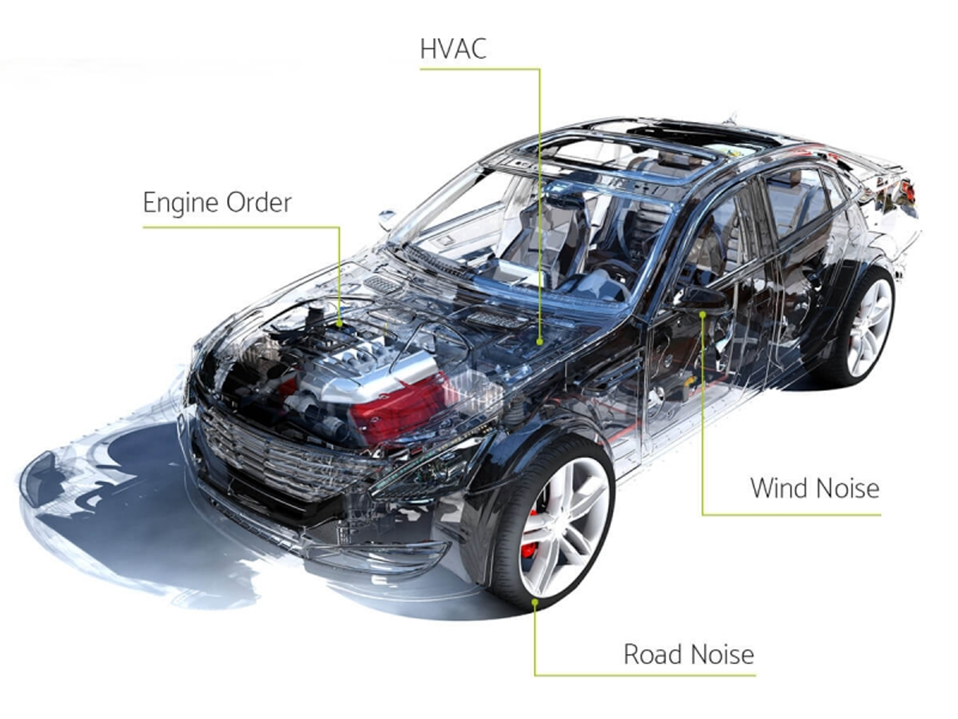
Here, use of AI in audio processing helps in creating a solution which significantly improves the Speech to Noise Ratio (SNR). The deep learning model is trained on multiple noise profiles with a variety of conditions and the resulting model can provide good results in suppressing noise and increasing the speech quality in varying environments.
Analyzing Road Conditions for Road Safety with the help of Smart Public Infrastructures
Connectivity has transformed the way in which human beings interact. The Internet has also enabled closer collaboration with machines, and smart public infrastructure systems are here to help achieve the overall goal of efficient, smarter, and safer cities.
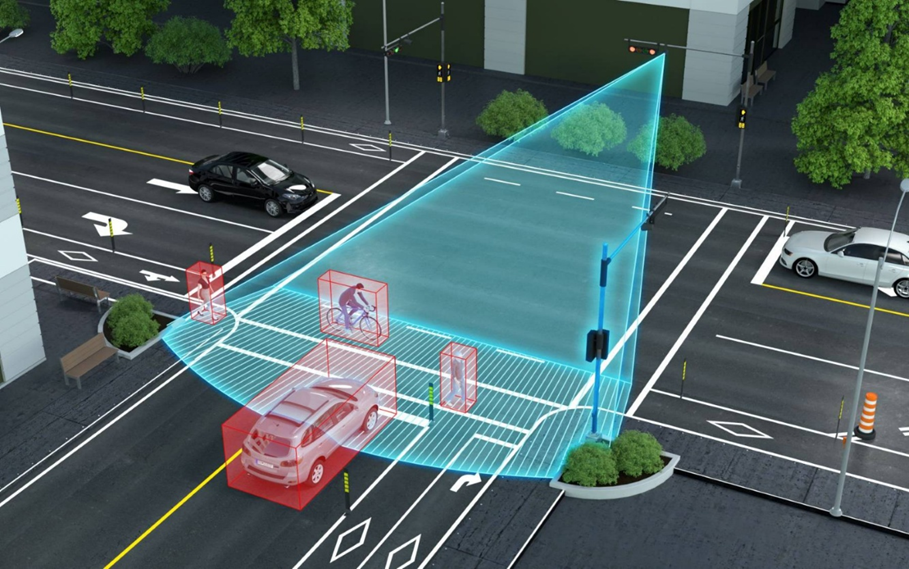
To make cities smarter, vehicles and infrastructure have intelligent systems that can monitor, measure, and analyze data in a highly dynamic environment. Such data is used to achieve smooth traffic flow, infrastructure management (example parking), pedestrian safety, people security etc. 3D Lidars are being tested instead of cameras, to sense traffic flow and send data to the cloud for multiple sink points, to help improve overall accuracy. Some examples are dynamic traffic signal management for smooth traffic flow and predictive road conditions as a service streamed to vehicles as an early warning which can then help the car/driver to anticipate road conditions and reroute their journey.
Expanding on the above, data from the cloud is streamed to the vehicle via cellular V2X (vehicle-to-everything) and is currently being tested with 5G connectivity due to its low latency. This enables Analyzing Road Conditions in real time mode.
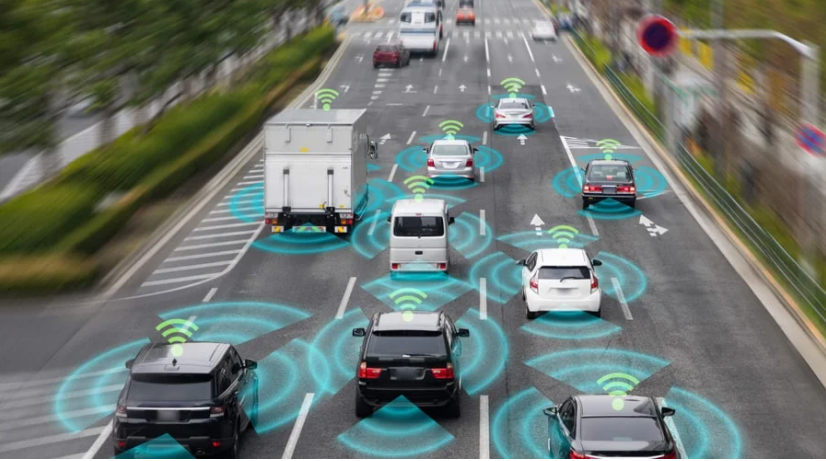
Real-time detection of road conditions offers continuous updates to drivers on road construction, vehicle crashes, monitoring speed limits and road closures prior to their journey. This is another area where AI-based predictive technology may prove to be extremely crucial for drivers to be able to gauge their routes and plan to avoid congestion or hazards.
Also, as the number of networked vehicles in the market increases, the vehicle data is streamed to the cloud and consumed by the car manufacturer for various purposes including data-set collection and predictive maintenance.
Conclusion:
It is clear from the use cases, some of which are listed above, that AI in Automobiles related to safe transportation, safety, and breakdown warnings, is mature and is here to stay.
European New Car Assessment Programme (Euro NCAP)* has always rewarded Occupant Status Monitoring (OSM) under the Safety Assist protocol that helps in decreasing behaviors and states linked to driver impairment. To get a full score in the OSM area, Direct Driver Monitoring system (DMS) will be a requirement from 2023 onwards as per the Euro NCAP roadmap (read here). Surely, DMS is ‘the next seatbelt’. And with the Euro NCAP’s 5 star rating, the period from ”paid-for-optional-features” to standardized technologies is shortened. Hence the automotive industry has been encouraged to move into a higher gear when it comes to offering these features as standards. Driver assistance and other safety systems have already made driving safer and more relaxed. The vision of ‘accident-free mobility’ in the automotive industry is turning into reality assisted by AI.
The deep footprint Ignitarium has in Product Engineering Services, and the current work that involves combining AI hardware, software, and services to create top-to-bottom automated mobility solutions, positions Ignitarium as a strong player and partner in this space.
* Euro NCAP’s five-star safety rating system help consumers and businesses to compare vehicles more easily and to help them identify the safest choice for their needs hence promoting standard fitment across the car volume sold in the European Community in combination with good functionality for these systems, where this is possible.