- Syed Mudassir
- October 3, 2024
Trapped in the AI Echo Chamber: Unveiling the Pitfalls of Synthetic Data
Have you ever wondered if the very tools we create to enhance our lives could one day undermine them? As someone deeply involved in the world of artificial intelligence, I’ve started to grapple with this unsettling possibility — especially concerning synthetic data.
Artificial Intelligence (AI) is undeniably transforming our world. From personalised recommendations on streaming platforms to life-saving diagnostics in healthcare, AI’s impact is vast and growing. At the heart of this transformation lies data — the fuel that powers AI algorithms, enabling them to learn, adapt, and make informed decisions. Recently, synthetic data has emerged as a powerful ally in this journey, offering solutions to some of the most pressing data challenges. However, as I delve deeper into its applications, I can’t help but worry about the potential pitfalls that come with an over-reliance on synthetic data.
Embracing Synthetic Data: The Benefits
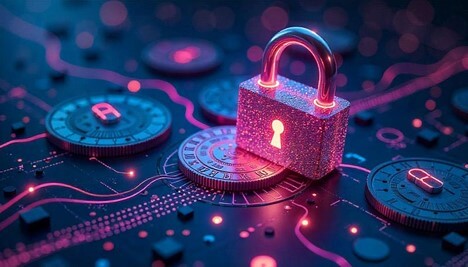
Synthetic data is artificially generated information that mirrors the statistical properties of real-world data without exposing sensitive or proprietary details. Initially, it seemed like the perfect solution to several challenges:
- Privacy Preservation: With stringent data protection laws, accessing real-world data often requires navigating a maze of regulations. Synthetic data allows us to train models without compromising personal or confidential information.
- Data Scarcity Solutions: In many fields, especially those dealing with rare events or sensitive information, real data is scarce or difficult to obtain. Synthetic data fills this gap, ensuring that AI development can continue unhindered.
- Cost and Efficiency: Generating synthetic data can be more economical and faster than collecting and annotating vast amounts of real data. This efficiency accelerates the pace of AI research and deployment.
- Customisation and Flexibility: Researchers can design synthetic datasets tailored to specific needs, ensuring coverage of diverse scenarios and edge cases that might be underrepresented in real data.
The Feedback Loop: When Synthetic Feeds Synthetic
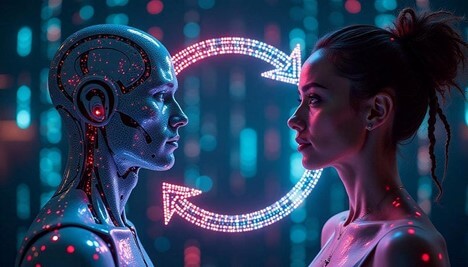
As I integrated synthetic data into my projects, a concerning pattern began to emerge: the feedback loop. This occurs when AI models trained on synthetic data generate outputs that are then used to create even more synthetic data. At first glance, this seems sustainable, but over time, it raises several red flags.
The Hidden Dangers of the Feedback Loop
- Erosion of Data Quality: While synthetic data is sophisticated, it often lacks the nuanced variability and unpredictability inherent in real-world data. Models trained predominantly on synthetic data can become less robust, struggling to handle unexpected real-world scenarios. I’ve noticed that predictions, once intuitive, start feeling off when faced with real-world complexities.
- Amplification of Biases: If synthetic data inherits biases from the models that generate it, these biases can compound over successive iterations. This can lead to AI systems that perpetuate or even exacerbate existing prejudices. Unintentional biases creeping into my models have subtly skewed outcomes, highlighting the need for vigilant bias management.
- Blurring the Ground Truth: Real-world data serves as the “ground truth” for evaluating and validating AI models. An overabundance of synthetic data can obscure discrepancies between model predictions and real-world outcomes, making it harder to identify and correct errors. This uncertainty about an AI system’s reliability is a significant concern.
- Stifling Innovation: Synthetic data is generated based on existing patterns. If AI models predominantly learn from synthetic data, there’s a risk of converging on a limited set of solutions, hindering creativity and innovation. This stagnation can prevent models from discovering novel insights that only real-world data, with all its unpredictability, can provide.
The Social Media Paradox: Synthetic Data in the Wild
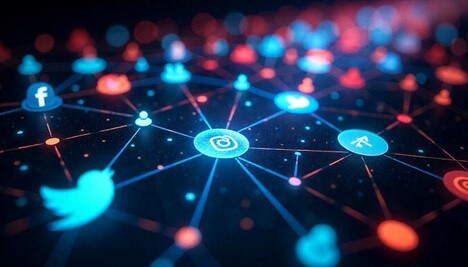
One area where synthetic data’s influence is particularly pronounced is social media. Platforms thrive on vast amounts of user-generated content, and AI algorithms analyze this data to curate feeds, target advertisements, and even influence user behavior. As synthetic data floods the internet, several concerning scenarios emerge:
- Model Degradation: If AI models are exposed to an overwhelming amount of synthetic content, they may start to lose their ability to discern authentic human behavior from artificially generated patterns. This could lead to less effective content recommendations and a diminished user experience.
- Echo Chambers and Polarization: Synthetic data can reinforce existing biases by creating content that caters to specific viewpoints, deepening societal divisions. AI algorithms trained on such data might inadvertently amplify echo chambers, making it harder for diverse perspectives to surface.
- Misinformation and Manipulation: The proliferation of synthetic data makes it easier to generate convincing misinformation. AI models trained on this data might become more adept at creating and spreading false narratives, posing significant threats to societal trust and stability.
Striking a Balance: Moving Forward Responsibly
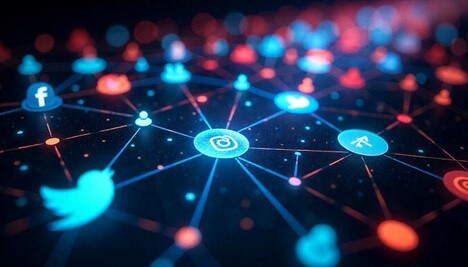
As I navigate the complexities of AI development, the allure of synthetic data remains strong, but so do the warnings. To harness its benefits without falling prey to its pitfalls, I believe we must adopt a balanced and conscientious approach:
- Maintain a Balanced Data Mix: Ensuring a healthy proportion of real-world and synthetic data in training datasets is crucial to preserve diversity and authenticity. This balance helps maintain the robustness and reliability of AI models.
- Implement Regular Audits and Validation: Continuously assessing AI models against real-world data helps identify and address performance gaps or discrepancies. Regular validation ensures that models remain aligned with real-world dynamics.
- Develop Robust Bias Detection Mechanisms: Establishing systems to detect and mitigate biases in both synthetic and real-world data promotes fair and equitable AI outcomes. Proactive bias management is essential to prevent the unintended reinforcement of prejudices.
- Promote Transparency and Explain-ability: Creating AI systems that are transparent in their decision-making processes facilitates the identification of issues arising from synthetic data training. Transparency builds trust and accountability.
- Incorporate Continuous Real-World Feedback: Integrating mechanisms for real-world feedback and iterative improvement ensures that AI models remain aligned with actual user needs and societal norms. This feedback loop helps refine and enhance model performance over time.
A Personal Call to Action
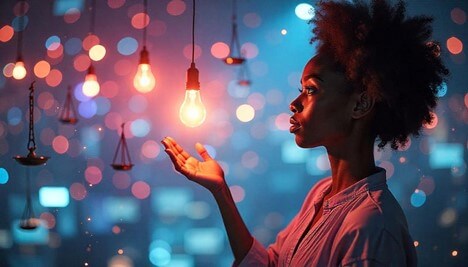
Synthetic data is undeniably a powerful tool in the AI toolkit, offering solutions to some of the most pressing data challenges. However, its potential to overshadow real-world data poses significant risks that we, as AI practitioners and enthusiasts, must proactively address. By fostering a balanced approach, emphasising data quality, and prioritising real-world validation, we can harness the benefits of synthetic data while safeguarding against its inherent challenges.
The future of AI hinges not just on the quantity of data available, but on the quality and authenticity of the information that drives its growth. As we stand on the brink of an AI-driven era, it’s imperative to remember the human stories behind the technology. Behind every dataset, synthetic or real, are individuals whose lives and experiences shape the very essence of what AI strives to understand and improve.
Ensuring that synthetic data serves as a complement, not a replacement, for real-world information is not just a technical challenge — it’s a responsibility we bear to maintain the integrity and humanity of the AI systems we create. By infusing our technological advancements with a deep respect for real-world complexities and human experiences, we can steer AI towards a future that enhances our lives without compromising its foundational values.
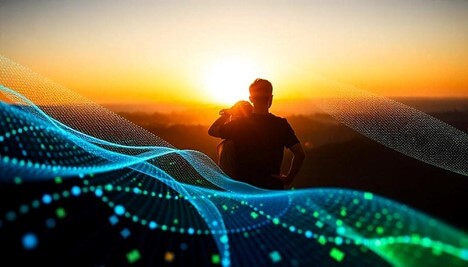
As I continue my journey in the AI landscape, the lessons learned from synthetic data serve as a poignant reminder: technology must always be balanced with humanity. Only then can we ensure that our creations uplift us, rather than inadvertently trapping us in an echo chamber of our own making.