- Athira M D
- February 2, 2022
Fall Detection & Warning System using Geofencing for Rail Passenger Safety
A fall detection system can be defined as an assistive mechanism whose main objective is to generate an alert when a fall event has occurred. There are many cases of train tragedies in which people fall from running trains or from railway platforms. Such an uncontrolled fall can result in critical injuries and can even lead to the death of the person. To reduce the adverse consequences of such unfortunate events, the need for efficient and intelligent systems to prevent and detect such events has significantly increased during the past decade. Many solutions, ranging from very simple systems to extremely complex ones, have been proposed considering different aspects of the fall detection problem.
The fall detection system discussed in this blog addresses the use case of people potentially falling off the edge of a regular railway platform. In this implementation, a standard surveillance camera monitors the region close to the platform’s edge and an AI algorithm running on an edge compute device acts as a fall detection and warning system.
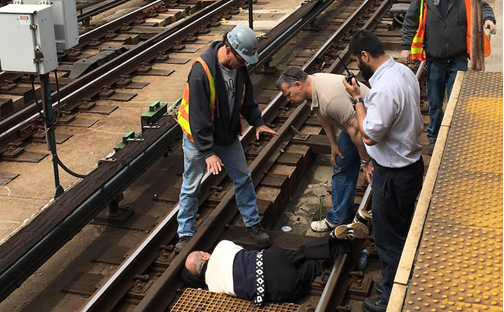
The main aim of this system is to manage risk at the platform-rail interface by efficiently providing alerts when a passenger goes inside this danger zone.
Data Preparation
Data preparation is the process of cleaning and transforming raw data prior to processing and analysis. It is an important step and often involves labelling data, reformatting data, making corrections to data and the combining of data sets to enrich data.
For this project, the data was collected by dumping frames from several CCTV cameras which captures footage of persons falling off the edge of a railway platform on to the rails. The labelling of data was done using the LabelImg tool with label annotations being captured as xml files.
Object Detection using YOLOv3
Object detection is a task in computer vision that involves identifying the presence, location, and type of one or more objects in a given frame. It is a challenging task that requires successful object localization in order to locate and draw a bounding box. YOLO is a family of deep learning models designed for fast object detection.
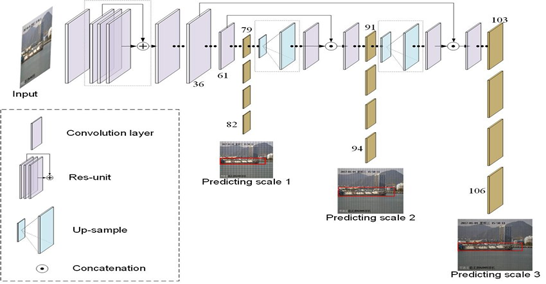
Fig 2: [Image Source]
The approach involves a single deep convolutional neural network which splits the input data into a grid of cells and each cell will directly predict a bounding box and its object classification, and a confidence score associated with it. The result is a large number of bounding boxes that are consolidated into a final prediction by using several post-processing steps like non maximum suppression. Compared to previous generations of YOLO models, YOLOv3 is much more powerful and contains the feature of detection at three different scales. More details regarding YOLOv3 can be found in the following links YOLOv3 and YOLOv3 Explained.
YOLOv3 uses Darknet as the backend. Darknet has a 53 layer architecture into which 53 more layers are added for the task of detection. Thus, this 106 layered network forms the consolidated architecture of Yolov3. The source code of YOLOv3 is available in the following open source link keras-yolov3.
Object Tracking using DeepSort
DeepSort (Simple Online and Realtime Tracking with a Deep Association Metric) is one of the commonly used object tracking algorithms. For tracking objects, we can feed the detections from Yolov3 into the DeepSort tracker [Link]. We use a Kalman filter for predicting the trajectory being tracked. Then cascade matching is done with the predicted trajectory tracks and detections in the current frame, and a filter is updated based on that. The following link DeepSort-Deep Learning applied to Object Tracking gives an overview of the algorithm.
Implementation of the Fall detection & warning system
The fall detection pipeline that was implemented in shown in Fig 3. It essentially detects and tracks the foot region of people on the platform and compares its position with respect to the ROI (region of interest) that represents the danger zone at the edge of the platform.
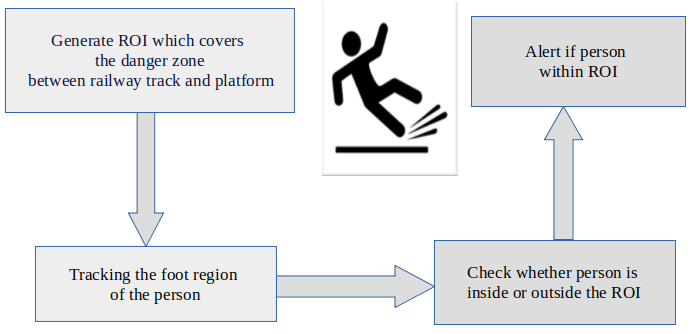
The implemented system is a UI based application, where the input to the application is a video feed. The UI system allows the drawing of the ROI which covers the danger zone between the railway track and railway platform. After generating the ROI, tracking is done of the people walking on the platform. The ROI acts as a virtual geo-fence. If the person’s foot region is inside the ROI representing the danger zone, the UI application will generate an alert indicating that the person is inside the risk area. The Yolov3 algorithm is utilized here for detection purposes and DeepSort algorithm is implemented for tracking the person’s foot region.
The implemented application is able to detect and track multiple people over user-creatable virtual geo-fence regions and can simultaneously detect multiple fall events in real time.
Conclusion
Deep-learning based person detection and foot tracking within virtual geo-fence regions representing physical risk areas, using low cost cameras and compute devices allow increased passenger safety on high footfall railway platforms.