- Radhakrishnan V and Vinay Kulkarni
- August 4, 2021
Building an AI-based Sound Event Classifier
Introduction
The term Sound Event Classification (SEC), also called Acoustic Event Classification or Audio Event Classification, is the process of acquiring audio signals from an audio source, analysing the acquired signals to detect events that we are interested in and if an event is detected, categorizing the event, which will be used to trigger actions by the downstream components or referred to consumers for further analysis and actions. As an example, law enforcement agencies can use the sound inputs from microphones attached to street cameras. An SEC system connected to the microphone feed can detect gunshots or human shrieks and can alert the authorities of an event of concern.
Sound Event Classification is increasingly gaining prominence in real-world applications. These applications span the domains of security, surveillance, medical / industrial diagnostics and many consumer devices. Technologies that revolutionised parallel processing using many compute cores (GPU/TPU) have catapulted the precision with which we can now detect occurrences of events using their acoustic profiles. Sub-systems of such nature tend to be a differentiator even in mainstream system designs that can detect, classify and react to events of impact.
Broadly, there are two different approaches to detection of events from audio signals. The first is a signal processing based method, which usually looks for a ‘template’ in the signal. The event is considered to have occurred if the input closely mimics the template. The second approach uses artificial intelligence as the engine to detect events from audio signals. Classical approach to Audio Event Classification relied on use of Machine Learning algorithms like HMMs (Hidden Markov Model) or modified versions of HMMs. However, their application and accuracy usually did not inspire confidence wrt reliability or effectiveness. With the onset of the deep learning era, the accuracy and efficacy of such systems saw considerable improvement, just by replacing the classical AI models with artificial neural networks (ANN). We observe that there is an increased adoption of ANN-based models. The remainder of this blog will focus on the design / architecture considerations of an AI-based solution which uses an ANN at the core of the event classifier.
Sound Event Classifier Design
An SEC application will have five stages –
1. Signal acquisition
2. Preprocessing
3. Feature Extraction
4. Classification
5. Post processing (optional)
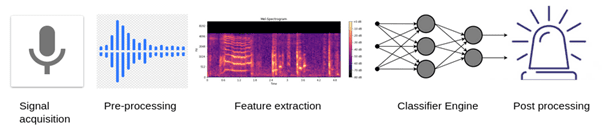
1. Signal acquisition – This is the stage when the audio stream enters the system. Based on the operating environment, a microphone has to be chosen with considerations involving directionality, frequency response, impedance and noise resilience. Wrong choice of microphone could affect the overall efficiency of the system. For example: if we choose a microphone with studio characteristics to be placed in outdoor systems, the wind noise could overwhelm the system, thereby rendering the downstream stages to be less effective. Another important design consideration would be the rate at which the analog audio signal is sampled.


Sampling rate or sampling frequency defines the number of samples per second (or per other unit) taken from a continuous signal to make a discrete or digital signal. Nyquist rule mandates the required sampling rate of a signal to be twice the largest frequency component of the signal, if we have to reconstruct the original audio from the samples. For example, the human ear can perceive audio signals in the frequency of 300 Hz to 20,000 Hz. By Nyquist rule, we will need to sample a signal at minimum rate (frequency) twice the highest frequency, i.e. at 40,000 Hz to attain theoretically perfect reproduction. A lower rate of sampling could cause an aliasing effect (incorrect representation of the original audio). Also, the sound event has to be studied closely before making a sampling rate decision. Pushing the sampling rate higher will not yield any improvement, instead it will add unnecessary processing overhead. Sampled audio signal is represented using numbers (quantized) and represented in binary format (encoded). Pulse Code Modulation (PCM) is a popular technique for quantizing and storing digital representation of audio signals as .wav files.
2. Preprocessing – Preprocessing of the audio signals is an important signal preparatory step. A few techniques used to prepare the signal for feature extraction are-
A. Filtering – This may involve passing the signal through one or more filters to ensure that the features we extract can be used by the classifier to classify the event. The acquired signal could have frequencies in all ranges. Often, the sound events that we are interested in could be in a short range compared to the spectrum supported by the microphone.
A beneficial side effect of filtering is noise reduction / removal. A study of the frequency range of the event often preludes the design of filters. The figure below is the frequency plot of the gunshot as represented in Fig B.
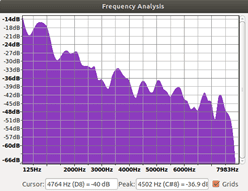
A software / hardware filter is implemented after careful consideration of the cost, efficiency and latency requirement of the system.
B. Activation / Trigger – In certain designs, a preprocessing phase may also include an activation mechanism for downstream stages. For example, a period of silence in an audio stream need not be passed through feature extraction or classification stages. This could help in reduction of processing cycles and hence, power consumption.In essence, a well designed preprocessing component helps to reduce the complexity of the system, remove or reduce the impact of ambient noise, lower resource consumption and cost.
3. Feature extraction – A machine learning / neural net classifier is ‘trained’ (exposed to) characteristics of an object or event to ‘predict’ the class it belongs to. This stage deals with techniques employed to extract these characteristics (‘features’). An audio signal can be characterised by its amplitude (loudness), frequency (pitch) and timbre (quality). Theoretically, each of these can be used as a feature, in practice, they are not used independently to classify any event. There are various techniques to extract signatures of the event. Short Term Fourier Transform (STFT), Mel Frequency Cepstral Coefficient (MFCC), LPC (Linear Predictive Code) are a few techniques commonly used to extract features from an audio signal. There are derivatives of these techniques that can improve the classification accuracy. Often, this is a stage which demands a relatively higher level of computation power. Hence, depending on the application and the associated constraints imposed by the environment or specifications, the designers may have to weigh on power consumption needs at this stage.
4. Classifier – A classifier can be a classical machine learning model (HMM) or a deep learning model (Artificial Neural Networks – ANN). An artificial neural network mimics the functioning of the human brain (a detailed explanation of concepts is beyond the scope of this blog. Readers are encouraged to refer to more articles available online / offline to gain deeper understanding of ANNs). Frameworks like Tensorflow, Keras and Pytorch are available in popular programming languages like Python/R or packages like Matlab. These frameworks enable us to define such networks, teach the network (referred to as ‘training’) with a set of samples, so it learns the patterns and eventually check the prediction accuracy ( referred to as ‘testing’ ) by passing some samples.
With the GPU revolution, a deep learning model based classifier is the norm rather than an exception. The network architecture will be heavily influenced by the constraints imposed by the availability of data to train the model, the operating environment, hardware / platform specification and the performance specifications. ANNs provide flexibility to system designers in determining the memory footprint, computational power and latency, thus enabling designs for tiny devices (IoT) to massively parallel systems that have been made affordable by Compute Cloud vendors. Compared to classical machine learning models, deep learning models need lots of samples to effectively learn the patterns. But, properly trained neural networks can enable prediction accuracy of more than 90%. Usually the neural architecture will be a series of convolutional layers followed by one or more dense layers.
Sample collection for training the model has to be given due importance. Though the deep learning models have an inherent strength to tolerate noise, the training samples should be selected carefully from the operating environment with all possible input combinations that we will expect the model to discern and classify. If there are not enough original data samples, synthesized data has to be introduced as training samples so that the network can learn different representations. This is referred to as data augmentation. To illustrate the utility of data augmentation, consider a gunshot sample acquired from an indoor shooting range. If we have to use this in a system to detect street events, this sample has to be mixed with ambient noise from the street. A firework display could cause a gunshot detection system to trigger a false alarm. Such events could lead to a false positive and the model has to be trained with such audio samples of confusing sound events to be immune to such events.
5. Post processing – This is an optional block in sound event classification and depends on use cases for an SEC. The follow-up action when an event is detected can be initiated in the post process stage. An audio signal is a continuous waveform in real life and digitisation changes its nature to a discrete sample representing a short time. If the system is designed to trigger actions when an event is detected, it is possible that the same event may be detected in adjacent samples, which is unintended. Continuing with the example of gunshot detection, it is possible that the same gunshot is detected in two audio frames and the application sends out two distinct alerts to the agencies. This has to be avoided. Post processing step should take care of such inadvertent consequences.
Other considerations: Compared to vision based applications, audio-based systems may be less intrusive and at lower (relatively) risk of violating privacy laws of the land. Nevertheless, the system designers have to factor in these considerations while deciding on the positioning of microphones and storing, using or distributing the information captured from these systems. Keeping an audit trail, or even better, including the necessary controls may help the organisation operating such systems to comply with laws and regulations. Accommodating these factors in the design, could save the vendor and customer organizations some effort.
The Ignitarium Advantage: At Ignitarium, we have developed AI-based audio event classifiers and voice command engines under the brand SeptraTM, targeting multiple operating environments. These solutions are designed to be deployable in a wide array of architectures ranging from embedded to Cloud based platforms, making it suitable for standalone deployment or in IoT environments. Ignitarium is a preferred ecosystem partner to top embedded system manufacturers with deep expertise in building solutions deployed on embedded platforms.
6 thoughts on “Building an AI-based Sound Event Classifier”
Установка видеокамер обеспечит защиту помещения круглосуточно.
Инновационные решения гарантируют высокое качество изображения даже при слабом освещении.
Вы можете заказать широкий выбор устройств, адаптированных для офиса.
установка камер видеонаблюдения частном
Профессиональная установка и консультации специалистов обеспечивают простым и надежным для каждого клиента.
Свяжитесь с нами, и узнать о оптимальное предложение в сфере безопасности.
Наш сервис поможет получить информацию о любом человеке .
Достаточно ввести никнейм в соцсетях, чтобы получить сведения .
Система анализирует открытые источники и цифровые следы.
глаз бога телефон
Информация обновляется мгновенно с фильтрацией мусора.
Идеально подходит для анализа профилей перед важными решениями.
Конфиденциальность и точность данных — наш приоритет .
Ответственная игра — это принципы, направленный на предотвращение рисков, включая поддержку уязвимых групп.
Платформы обязаны предлагать инструменты контроля, такие как лимиты на депозиты , чтобы избежать чрезмерного участия.
Регулярная подготовка персонала помогает реагировать на сигналы тревоги, например, частые крупные ставки.
сайт вавада
Предоставляются ресурсы горячие линии , где можно получить помощь при проблемах с контролем .
Следование нормам включает проверку возрастных данных для обеспечения прозрачности.
Задача индустрии создать безопасную среду , где удовольствие сочетается с психологическим состоянием.
Ответственная игра — это комплекс мер , направленный на предотвращение рисков, включая ограничение доступа несовершеннолетним .
Сервисы должны внедрять инструменты контроля, такие как временные блокировки, чтобы минимизировать зависимость .
Обучение сотрудников помогает реагировать на сигналы тревоги, например, неожиданные изменения поведения .
https://sacramentolife.ru
Предоставляются ресурсы консультации экспертов, где обратиться за поддержкой при проблемах с контролем .
Соблюдение стандартов включает проверку возрастных данных для предотвращения мошенничества .
Ключевая цель — создать условия для ответственного досуга, где риск минимален с психологическим состоянием.
Осознанное участие — это минимизирование рисков для участников, включая установление лимитов .
Важно планировать бюджет , чтобы сохранять контроль над затратами.
Используйте инструменты временной блокировки, чтобы ограничить доступ в случае потери контроля.
Поддержка игроков включает горячие линии , где можно получить помощь при проявлениях зависимости .
Участвуйте в компании, чтобы избегать изоляции, ведь семейная атмосфера делают процесс более контролируемым .
авиатор играть
Проверяйте условия платформы: сертификация оператора гарантирует защиту данных.
При выборе компании для квартирного перевозки важно проверять её наличие страховки и репутацию на рынке.
Изучите отзывы клиентов или рекомендации знакомых , чтобы оценить надёжность исполнителя.
Уточните стоимость услуг, учитывая объём вещей, сезонность и дополнительные опции .
https://kuplukvartiru.com.ua/forum-nerukhomosti/remont-stroytelstvo-dyzain/14765-hruzchyky-plius-usluhy-hruzchykov-v-kyeve-y-po-vsei-ukrayne#38217
Убедитесь наличия гарантий сохранности имущества и запросите детали компенсации в случае повреждений.
Оцените уровень сервиса: дружелюбие сотрудников , гибкость графика .
Проверьте, есть ли специализированные автомобили и упаковочные материалы для безопасной транспортировки.