MLOps – ML Production Revolution
This blog is to stress the importance of MLOps in the ML project lifecycle, assert the necessity of MLOps in the context of the entire Software industry, explain its relevance within the context of DevOps, and how the changing Software development environment and processes help the ascend towards the peak of the Digital Revolution.
MLOps – What’s that got to do with Production or Revolution?
To understand this relation, we need to go through a bit of history and some examples of how inventions and innovations revolutionized the technology industry, which has, in turn, revolutionized several other industries. We’ll put this in the context of Machine Learning and MLOps, and explain the rationale behind the necessity to put more effort and focus into it.
There are many jargons, acronyms, noise, and clutter around this space that decision makers are finding it hard to come to a conclusion on when and where to invest.
The top 3 questions and concerns that we hear from business stakeholders when we pitch the importance of MLOps are:
- MLOps seems to be much more intense than the Development Operations we have in house. Why can’t this be integrated to the existing DevOps cycle?
- Can we consider MLOps once after we vet the success of ML projects or ideas?
- Cost of implementing MLOps right away might outweigh the budget for the ML PoC or idea. How can we justify this additional spending?
This article aims to alleviate these concerns and make these questions self-explanatory by stressing the importance and necessity of MLOps.
To understand the significance of the term “production” in the title, let’s delve a bit into the history of the Industrial Revolution. This will provide the context for the argument.
The first industrial revolution started with water and steam, and created a disruption in how mechanical production happened. Steam powered machines and machine tools revolutionized how tasks were accomplished, and how people got from one place to another. This was the beginning of the factory, and the transition to new manufacturing processes.
The technological revolution saw rapid progress in science, applied science and technology in mass production. Factories, production lines and processes vastly improved with the advent of gasoline engines.
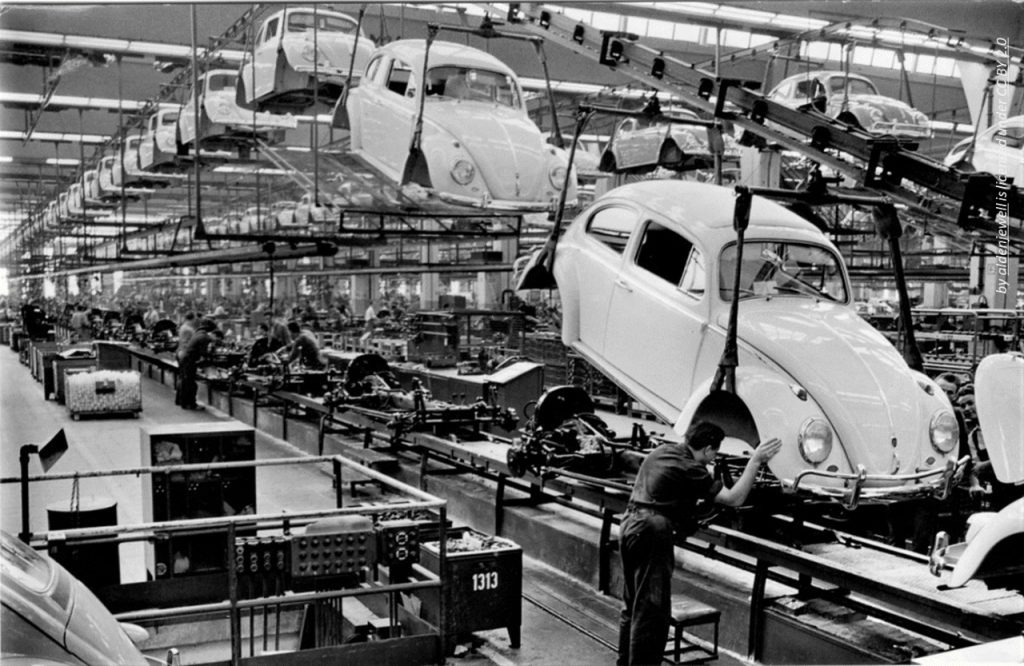
The digital revolution – saw groundbreaking inventions happening with semiconductors, Integrated Circuits (ICs), miniaturization, microprocessors, affordable computing device form factors, wireless technologies, internet etc. This was the beginning of the Information age and most importantly this was the time when Software came to be recognized as a product, and this outlook changed the entire dynamics of this age.
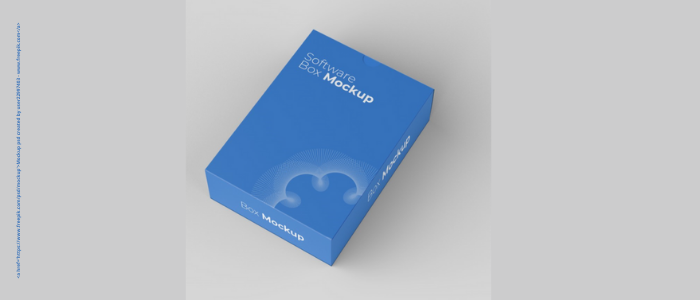
The exponential growth in the digital age happened due to Software. Once we arrived at a point where we were able to create the right kind of generic computing hardware for the software to shine on, things started moving at an exponential pace.
Every industry has to write code and program their systems in some form or the other. Every device / equipment that you see in any domain is heavily software driven. Take for e.g. EDA, CAD/CAM, Physical design tools, hardware description languages like VHDL or Verilog, AV workflows, broadcasts, content delivery, just to name a few. Hardware and Chip design, development & manufacturing workflows are heavily software driven, and this led to much improved platforms to run even better Software, which led to an optimizing cycle.
Emergence of a common theme
If closely analyzed, we can see a pattern emerging across all the industrial revolution phases. Each one starts with mechanization and automation using new discoveries or inventions. But, across each phase we see a period of drastic improvement to the factory and production line prevalent during that phase. These factory and production line advancements lead to the pinnacle of each phase, until they are completely surpassed by a totally new invention.
For example, it was the James Watt steam engine that upraised the first phase, the internal combustion engine revolutionized the second, and now Software is changing the third. Since the current digital phase is primarily Software driven, this summit can only happen through advancements in Software development and delivery process. That is, the very Software factory that produces Software. This has to span across industries and domains.
The way we do Software Development now is vastly different from what was the norm 10 years ago. Alongside that we are also witnessing the emergence of new Software development paradigms like Deep Learning helping us reach new areas and fill gaps, which we thought would never be possible with Software. Let’s now talk about this new Software paradigm and the improved Software factory and production lines.
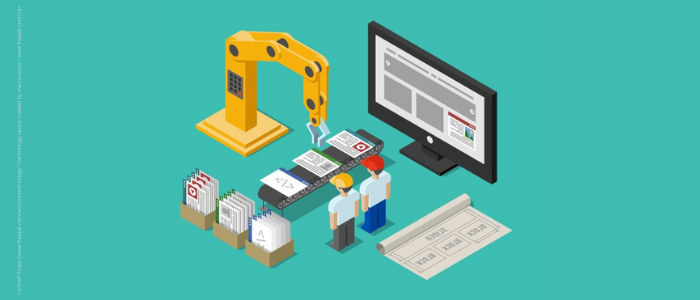
Enter the DevOps Era
https://en.wikipedia.org/wiki/DevOps
DevOps is now considered a standard practice with or without its knowledge! Development teams across the globe have started the practice knowingly or unknowingly! It is slowly turning out to be the de facto way of Software Development and Delivery. There is still a long way to go before DevOps gets fully adopted, and the industry as a whole is starting to benefit from the rapid pace and quality of Software.
DevOps is not a single person’s job or a single team’s job. It’s not a Job title, because it is not a job function, role, or technology per se. It is a collaborative methodology for doing Software Development and Delivery. Over the past few years the amount of quality tooling, processes, and workflows that got introduced to the development and production line, have made significant improvements to the way Software is produced and delivered. As noted earlier, mostly everyone in every industry is writing Software one way or the other, and this trend is going to go up exponentially.
Machine Learning, a new Software paradigm
In the Artificial Intelligence parlance, there are different approaches like Symbolic, Deep Learning, and hybrid ones like the IBM Watson. When we use the term AI, ML or Deep Learning in this article, we mean “Supervised Learning”, which is one form of Deep Learning. Other AI and ML approaches might need radically different computing platforms, development, delivery and operational workflows which is beyond the scope of this article.
Supervised learning is basically learning an X -> Y mapping, a function that maps an input to an output based on example input-output pairs.
With the supervised learning approach there is a paradigm shift happening with how we program computers, and basically this is changing our relationship to computers from a development and usability perspective. Instead of programming computers, the ML approach is to show them, and let them figure it out. That’s a completely different way of developing Software, when whole industries are built around the idea of programming computers. Educational institutions and Corporations are only now slowly catching up to this paradigm shift.
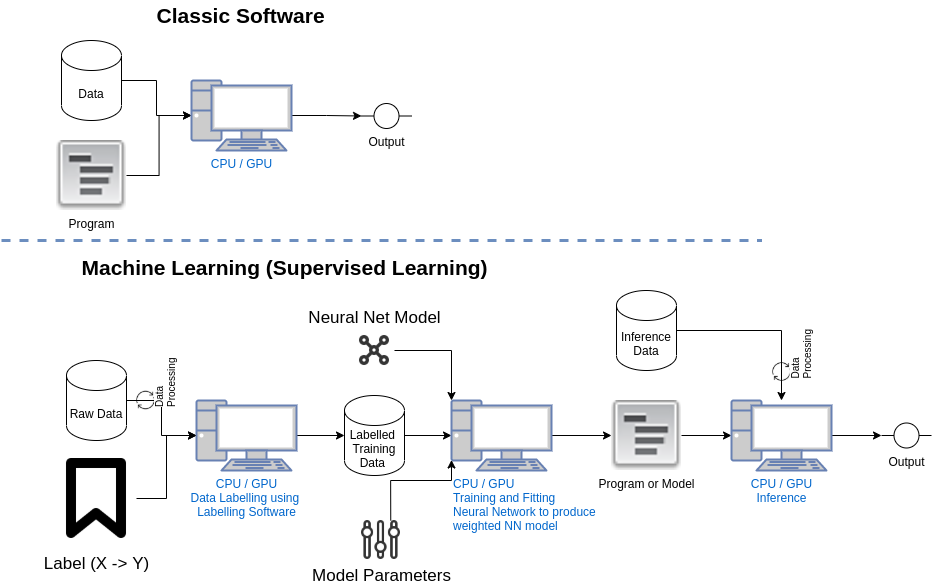
So what needs to be shown, and what is there to figure out
In simple terms, we need to show the data and the mapping (X -> Y), and what is being figured out is an approximate function for the mapping. X denotes the feature vectors from the input data and Y can be thought of as the labels for the ground truths. The approximation functions are figured out using back propagation algorithms like Gradient Descent, Momentum, Adam, RMSprop etc. These algorithms continuously try to optimize the weights and bias factors by minimizing the cost or loss function for the entire training set. The goal is to identify weight factors that make the convex cost function go down the slope, and reach the global optima as quickly as possible. This should be optimized for the entire training sample. This is a mouthful, but in very simple terms you need to show the data and mapping or labels to the computer, and the algorithms will learn this mapping as an approximation function, which we can later use for prediction.
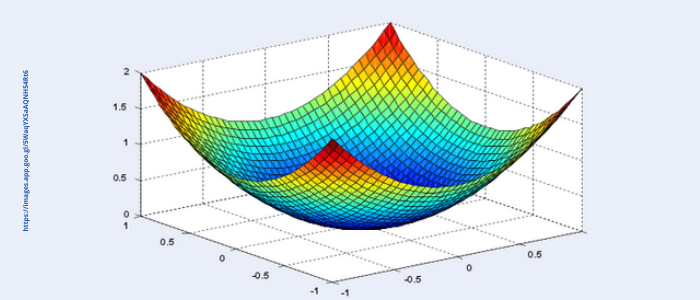
But, how does this actually work?
Nobody clearly knows, and people have drawn analogies to how the brain learns etc. which is a bit far-fetched imagination.
But the fact is, this “forward-prop” & “back-prop” method used in Supervised Learning has turned out to be a very good way for finding an approximation function, and this function will work quite well, subject to certain conditions. These conditions form the pillars for our discussion forward. Recently researchers and big internet companies have shown us that supervised learning has worked brilliantly well especially with some unstructured use cases, like image, audio, video, speech, NLP etc. where traditionally computer algorithms (expert systems) were not that good.
Deep supervised learning is a very empirical and iterative process and by “empirical process” we mean – you just have to try a lot of things and see what works. There is no magic bullet! Remember we’re trying to learn an approximation function. Since this is entirely data driven, it will always be evolving. Data is the fuel here. For compliance reasons, even the explanation for a prediction or result from a neural network model needs to be done in data parlance.
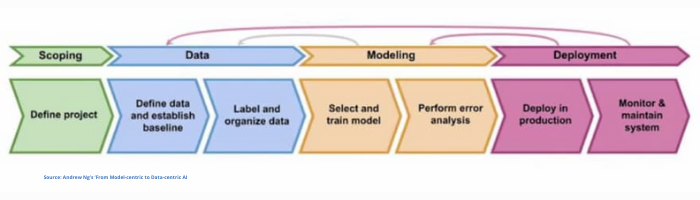
TL;DR: What you need to show the computer is a lot of good data and mapping (labels), and what they are figuring out is an approximation function.
So, isn’t this Software?
Yes, it is Software, and it also needs tons of typical software and hardware around it to work. It’s a different way of programming, the key factors being…
- Data is the fuel here
- Labelling is the labor here
- Experimentation is the process here
And maybe we can also add this…
- Weaving networks differently is the research here
All of this is very much iterative & empirical in nature, much more than typical SDLC (Software Development Lifecycle). The empirical nature of the Model Development Life Cycle is much more of a necessity than typical SDLC. This cycle needs to happen at a much more agile pace, needs to be monitored & measured continuously, can never stop because of data evolution or seasonality, needs to be sensitive, needs to be responsible and the list goes on. It’s not like typical Software processes don’t need any of these; In ML this is a necessity. So hope you are getting a sense of where we’re going with this, and why we’re pitching the importance of MLOps to keep all of this running and improving. Please keep reading as the pitch will be more clear and evident after some more points.
Analogy to make the ML paradigm apparent
When we talk or write in English, do we always think and apply the rules of the English grammar? I am a non-native English speaker and I never learned English grammar by the rule-based approach (symbolic approach)! Then, how do we speak or write without explicitly studying or knowing any of these? It’s because the brain might have heard, read, and got trained, and developed some sort of an approximation strategy. More experience means more data, and the better we get without explicitly knowing all the rules.Page Break
This is a very crude analogy of supervised learning and one shouldn’t draw analogies to the human brain functions from this example. Consider ML as naive neuroscience, just like genetic algorithms are naive biology! 🙂
So what’s MLOps then?
If you’ve come this far, you might have understood that we need tons of good data, continuous monitoring, continuous training, and continuous experimentation, or in short continuous operations to make ML work. It’s empirical, iterative & data centric by nature. There is no fixed forward function which you know upfront how to program! You don’t know that function, so you need to derive approximation functions using forward-, back-prop techniques, and for that you need to train/fit the neural network model with data & labels. But the world (data, labels, truths) evolves, and therefore for the function to stay relevant the entire cycle of operations (data collection, mapping, labelling, feature engineering, training, tuning etc.) needs to churn along. This is a necessity in the case of ML projects! Learning and adapting continuously is the simple mantra behind successful Deep Learning or ML projects.
This operational cycle is called “MLOps”. Without this optimizing cycle there is no relevance for ML projects or ideas.
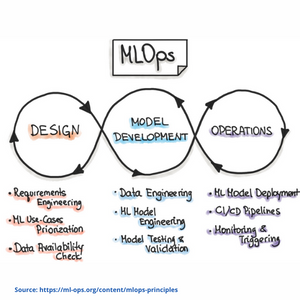
MLOps is not a single person’s job or a single team’s job. It shouldn’t be used as Job title, because it is not a job function, role, or technology per se. It is a collaborative, iterative, empirical, and data centric methodology for doing Machine Learning Model Development and Delivery.
Stressing the Significance of ML projects and MLOps
To understand the significance of ML projects and therefore MLOps, we’ll take a slight detour. Earlier we talked about two fundamental approaches, programming functions and learning functions. Some questions arising here are…
Will the two fundamental programming paradigms coexist? Or will it replace the Software expert systems, symbolic representations, and the traditional Software development in general?
Proponents of ML have argued the need for more people who get computers to do things by showing them. Large corporations like Google are now training people, called brain residence. A lot of new aspiring engineers actually want to work on ML.
But, both these approaches are going to coexist in the future. Even in the AI space the symbolic and non-symbolic representations will co-exist. This coexistence is necessary because…
- There are certain areas where the classic approach shines.
- There are other areas where classic approaches lack, especially the unstructured ones, like vision, NLP, translations, object detection, image classification etc. where the Human Level Performance outshines. ML will fill this gap.
- There are cases where we still don’t know, or have not developed the function to program. So we need to learn those functions from existing data and labels.
- There might be yet other use cases where ML approaches may be used to start with, and then these networks and their connections and weights will be analyzed and studied to deduce a generic function.
In fact if you see it from another angle, the feature engineering and feature extraction work that is given much importance in supervised learning can be thought of as a step towards designing an expert or classic system. Data scientist / domain expert studies, analyzes the data to extract the features that they think might have significant impact on the results of the model. It should be clear by now, why MLOps is called a “data centric” methodology. What makes MLOps a necessity is the empirical, iterative, and data centric nature of supervised machine learning to sustain its relevance. This makes MLOps much more intense than the typical DevOps cycle we are used to.
Both Software and ML development & release workflows are super essential for revolutionizing the grand Software production line. Efficient DevOps and MLOps practices and tooling will be key to climb the summit of the Digital Revolution. This is true for all industries and all domains.
What if there is no proper MLOps?
By this time, you might have already understood the issues with not having a production line workflow and tooling for ML projects and software projects in general. There are many facets to it, but we’ll briefly touch upon some of the most important ones.
Lack of proper experimentation tracking – will lead to chaos for data scientists and ML engineers, and is a recipe for underperforming Models in production. As we’ve repeated throughout the article, supervised ML is a highly iterative, data intensive, highly empirical process. If there aren’t enough tooling and processes to track and analyze these experiments during development and operations, it’s simply not going to work.
Model irrelevance – will be the direct result of not having an end to end streamlined MLOps workflow. For ML projects, the most important mantra to remember is that the real development starts with the first deployment. When the model starts to see the real world data, it’s going to be a different story altogether. If you didn’t have a process or workflow to monitor the model performance w.r.t input and output metrics, measure the drifts, detect outliers, or if you didn’t have a way to collect and ingest real data back into the workflow, and retrain the model, the model is not going to stay relevant for long. This will have a direct impact on the business if it’s relying on this model for its core operations. So MLOps shouldn’t be an afterthought, it should be set up right alongside the first lines of code or data that you develop or collect.
Cost effectiveness – should be top priority for any ML project. Afterall primary aim for any ML project is to meet or even exceed Human Level Performance (HLP). This is true for use cases which humans were typically good at, and also for those tasks where humans typically relied on computers. The main fuel here is data, mapping, features that can be engineered out of the data. The cost impact of these resources and processes is a very important question. For that 0.1% improvement, if it’s going to drain the pocket by an additional 10%, does it make any sense? This could be development or operational costs like infrastructure scale cost, data storage costs, data transformation costs, training compute costs, inference costs, specialized hardware cycles etc. Without MLOps processes, tooling, and workflow there’s no way to measure and quantify these metrics and act upon them in an iterative manner. Without MLOps there’s no way to identify whether an ML idea is worth pursuing, and quantifying its cost effectiveness.
Implementing MLOps is definitely going to cost an organization for the tooling, infrastructure etc. But the long term benefits and savings it brings about far outweighs its cost. Over time, organizations should develop, unify, and enforce standard MLOps tools & practices across many ML projects and teams. This is key to avoiding disasters down the line.
Conclusion
Without continuous Development and Operations, there is no relevance for ML projects. It’s so much more critical for ML projects due to its nature. MLOps tooling, workflows, processes, pipelines, etc. should be set up right alongside experimentation of the project idea. Common MLOps guidelines, infrastructure, tooling environment etc. across multiple projects in an organization is also critical for streamlining and cost reduction. Think of supervised deep learning as one big Continuous Experimentation, a Lab that runs forever. MLOps is only the way to tame this highly iterative, empirical, data intensive genre of Software development. Every industrial revolution started with new discoveries and paradigm shifts in production, and then reached their summit by advancements in these production lines. We see this grand DevOps space with MLOps being an integral part of it, as the cornerstone for production line optimization which will take the current Digital phase to its pinnacle.
In the next set of blogs, we’ll delve deeper into what needs to be done differently, pipelines, lineage, provenance, experiment tracking, benchmarking, continuous integration / continuous deployment / continuous monitoring / continuous training, responsible data handling, how tools can help streamline the process, how much automation is good, and much more. So stay tuned…